More Bi-Directional Path Tracing
November 6, 2013 - 4:13 pm by Joss Whittle C/C++ GPGPU Graphics Java L2Program PhD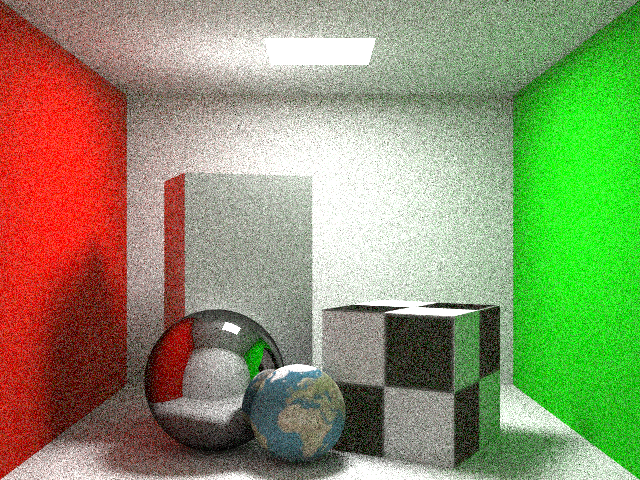
It’s still not perfect, far from it in fact, but it’s progress none the less. I’ve been reading a lot lately about Metropolis Light Transport, Manifold Exploration, Multiple Importance Sampling (they do love their M names) and it’s high time I started implementing some of them myself.
So it’s with great sadness that I am retiring my PRT project which began over a year ago, all the way back at the start of my dissertation. PRT is written in Java, for simplicity, and was designed in such a way that as I read new papers about more and more complex rendering techniques I could easily drop in a new class, add a call to the render loop, or even replace the main renderer all together with an alternative algorithm which still called upon the original framework.
I added many features over time from Ray Tracing, Photon Mapping, Phong and Blinn-Phong shading, DOF, Refraction, Glossy Surfaces, Texture Mapping, Spacial Trees, Meshes, Ambient-Occlusion, Area Lighting, Anti-Aliasing, Jitter Sampling, Adaptive Super-Sampling, Parallelization via both multi-threading and using the gpu with OpenCL, Path Tracing, all the way up top Bi-Directional Path Tracing.
But the time has taken it’s toll and too much has been added on top of what began as a very simple ray tracer. It’s time to start anew.
My plans for the new renderer is to build it entirely in C++ with the ability to easily add plugins over time like the original. Working in C++ gives a nice benefit that as time goes by I can choose to dedicate some parts of the code to the GPU via CUDA or OpenCL without too much overhead or hassle. For now though the plan is to rebuild the optimized maths library and get a generic framework for a render in place. Functioning renderers will then be built on top of the framework each implementing different feature sets and algorithms.